The Key Benefits of Implementing a Healthcare Interoperability Platform
Learn the key benefits of a healthcare interoperability platform. Improve patient outcomes and streamline operations. Click to read the full article!
- Date
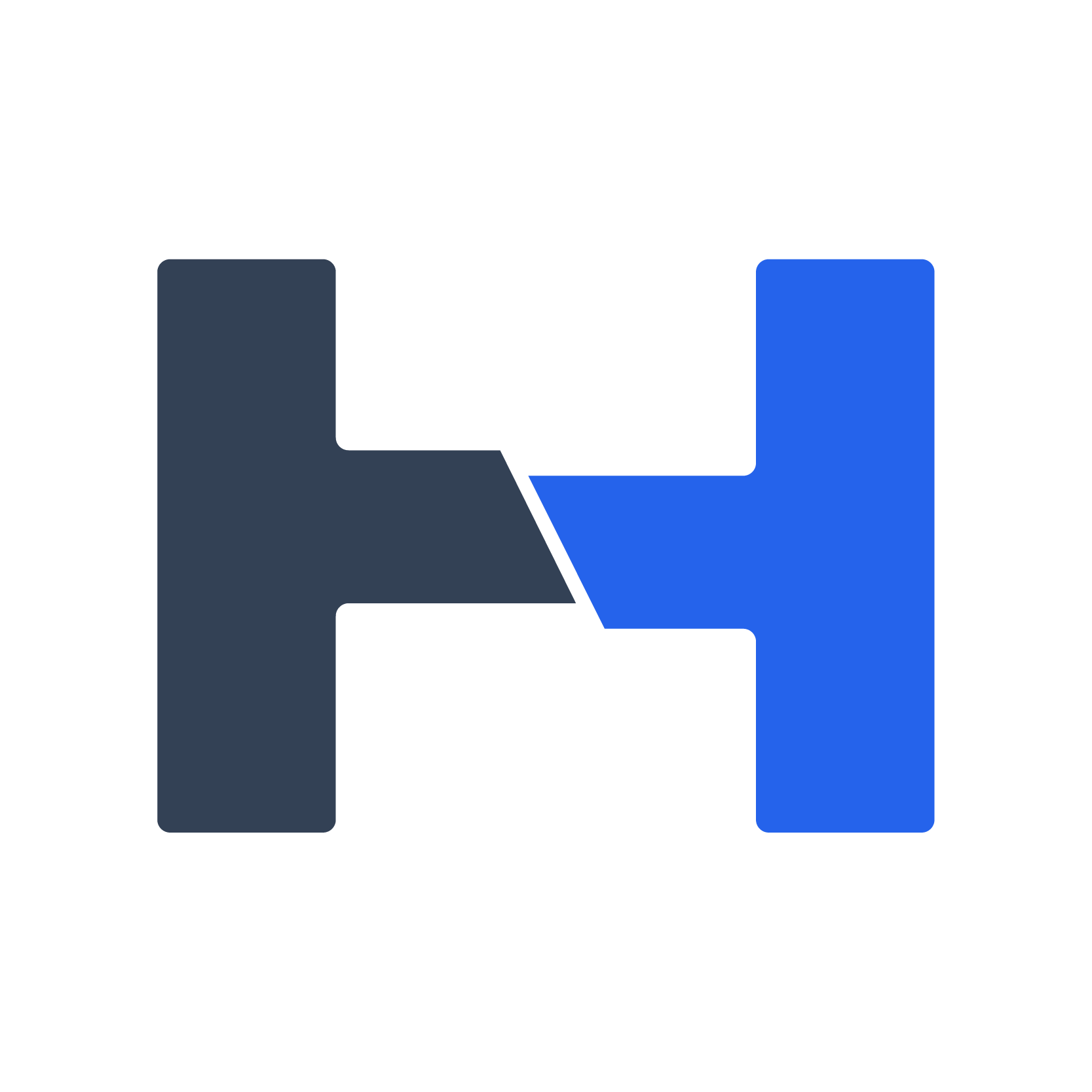
Iron Bridge
Learn about the latest news and posts from the Iron Bridge team.
Learn the key benefits of a healthcare interoperability platform. Improve patient outcomes and streamline operations. Click to read the full article!
Iron Bridge
Find out how electronic lab reporting software is enhancing healthcare data management processes. Explore our blog to learn more!
Iron Bridge
Want to improve your healthcare IT systems? Discover top strategies and enhance efficiency. Click to explore and read now!
Shane Smith
Director of Operations
Explore key methods for seamless healthcare data integration. Improve efficiency and outcomes! Get insights on our blog now.
Brian Brandebura
Co-founder/ COO
Discover the critical roles clinical pharmacists play in vaccination efforts. Learn their duties and impact. Read our comprehensive guide now!
Jaime Coll
Onboarding Project Manager
What are the benefits of vaccine management software? Discover the key features for streamlining your processes. Learn more today!
Darrell Crabtree
Director of Customer Success
We're excited to share that Iron Bridge will be at the Healthcare Information and Management Systems Society (HIMSS) conference in Orlando, FL. This premier event is an essential gathering for healthcare professionals, where the future of healthcare technology is shaped through collaboration and innovation.
Jaime Coll
Onboarding Project Manager
The Office of the National Coordinator's New Interoperability Rule and its Relationship to EHR Mandates
Darrell Crabtree
Director of Customer Success
Iron Bridge Corp ("Iron Bridge"), a leading provider of healthcare data interoperability solutions, announced the production availability of support for Fast Healthcare Interoperability Resources version 4 ("FHIR R4") in its most recent healthcare data exchange Application Programming Interface ("API") through its Nuvola Hub Healthcare Integration Platform as a Service ("Hi-PaaS").
Jesse Reynolds
Chief Architect
Streamlined submission to public health agencies to improve clinical outcomes and cash flow for ambulatory practices.
Brian Brandebura
Co-founder/ COO
Pharmacies in California are now mandated to submit vaccinations to the California Immunization Registry (CAIR).
Jaime Coll
Onboarding Project Manager
ADP® AdvancedMD®, a leader in all-in-one, cloud electronic health records (EHR), practice management, medical scheduling, medical billing services as well as a pioneer of big data reporting and business intelligence for smaller medical practices, today announced a new partnership with Iron Bridge Integration (IBI).
Brian Brandebura
Co-founder/ COO
Iron Bridge Integration has decided to allow interested EHR vendors and hospitals to utilize PubHub at no cost with one provider, practice, or hospital. We are confident in PubHub’s ease of use and simplicity and are currently in the process of signing up providers already taking advantage of this opportunity.
Brian Brandebura
Co-founder/ COO
Net Health, the leader in software solutions for specialized outpatient care, is partnering with Iron Bridge Integration to support clients' efforts in achieving Meaningful Use requirements related to electronically transmitting immunization records to state agencies.
Brian Brandebura
Co-founder/ COO
CareCloud, the leading provider of cloud-based practice management, electronic health records (EHR), and medical billing software and services, today announced it has partnered with Iron Bridge Integration (IBI) to leverage Pub Hub, IBI's public health clearing house.
Brian Brandebura
Co-founder/ COO
Iron Bridge Integration (IBI) announces the general availability of "Pub Hub", the healthcare industry's first public health clearinghouse.
Brian Brandebura
Co-founder/ COO
Iron Bridge Integration (IBI) is launching NuvolaHub, a platform that helps healthcare providers such as physician practices, ambulatory clinics, hospitals and other healthcare organizations solve the problem of exchanging data.
Brian Brandebura
Co-founder/ COO